Abstract:
Understanding the formation of stars and planetary systems is essential to answering many foundational questions in astrophysics. To address these questions, we rely on advanced numerical simulations and data from next-generation telescopes such as JWST and ALMA. In this talk, I will discuss how Machine Learning (ML) models can bridge the gap between traditional physics-based frameworks and data-driven methods, driving new progress in astrophysical research. In the first half, I will introduce Disk Planet Neural Network (DPNNet), an ML model designed to detect exoplanets in protoplanetary disk images. DPNNet represents a breakthrough in observational astrophysics by integrating Bayesian and other probabilistic ML methods to quantify uncertainties—a critical step for accurate data interpretation. In the second half, I will present Gravity-Informed Neural Network (GRINN), a Physics-Informed Neural Network (PINN) designed to solve 3D self-gravitating hydrodynamic systems. With GRINN, we achieve approximately 10x reductions in runtime compared to traditional grid-based methods for solving systems of partial differential equations (PDEs) in 3D. Together, these models demonstrate the potential of Scientific ML (SciML) to develop next-generation numerical solvers for astrophysical modeling, paving the way for AI-driven advancements in astrophysics.
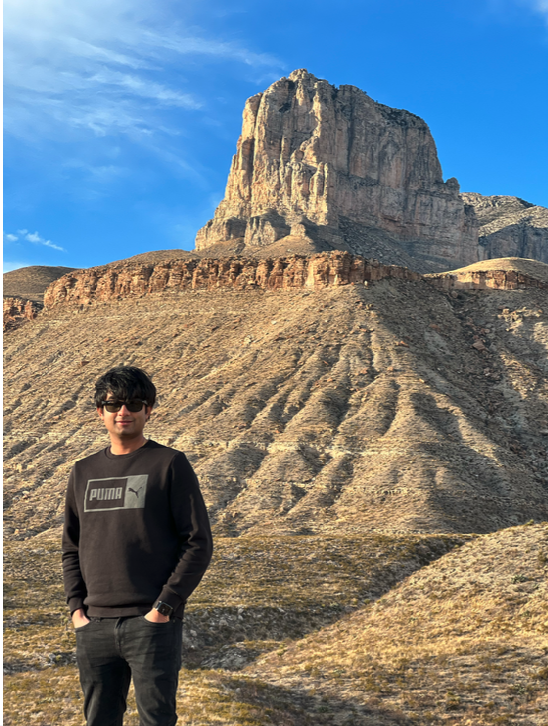